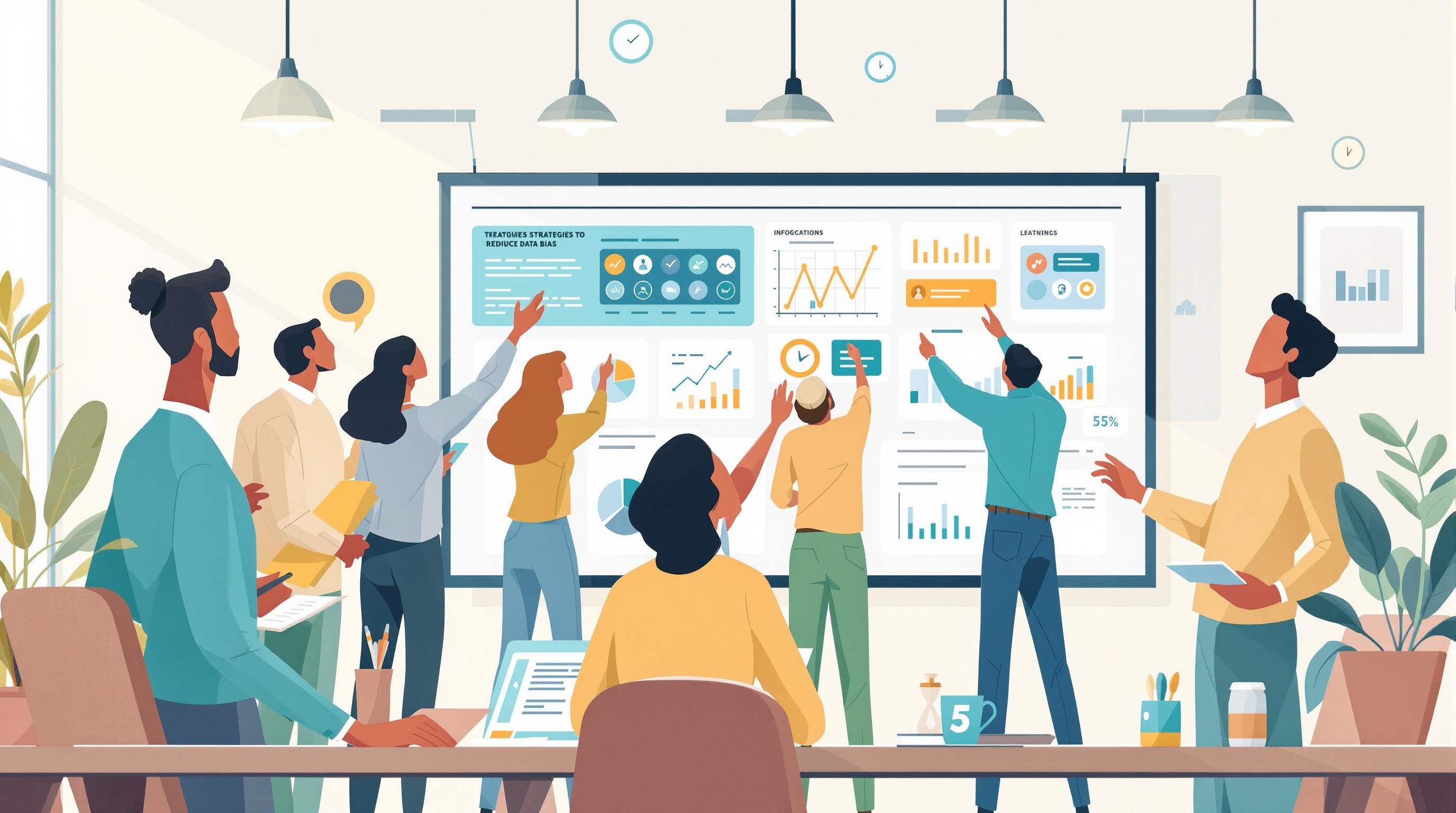
5 Ways to Reduce Data Bias in Infographics
Want to make your infographics more accurate and reliable? Here's how to reduce data bias effectively:
- Use Diverse Data Sources: Rely on multiple credible sources to avoid skewed perspectives.
- Verify Data Accuracy: Cross-check for reliability, check sample sizes, and ensure relevance.
- Add Proper Context: Include timeframes, demographics, and methods to clarify the data.
- Choose the Right Visuals: Pick charts that represent data truthfully without distortion.
- Review for Bias Regularly: Test visuals with diverse reviewers and track audience feedback.
Quick Tip: Avoid common pitfalls like cherry-picking data, manipulating scales, or omitting key context. Use tools with built-in bias detection to streamline the process.
Want to dive deeper? Let’s break down each strategy step-by-step.
Related video from YouTube
What Is Data Bias and How Does It Affect Infographics?
Data bias refers to consistent errors in how information is gathered, analyzed, or displayed, which can lead to misleading conclusions [1][2]. When this happens, it undermines the reliability and effectiveness of data-driven visuals.
Take this example: In 2023, a tech company released an infographic claiming a 40% increase in diversity. However, the data only reflected their headquarters, leaving out global offices where diversity metrics were lower [1][3].
In infographics, data bias often appears in three main forms:
1. Collection Bias: This occurs when the data collection process leaves out specific groups. For instance, relying only on social media surveys excludes those who aren’t online [1][2].
2. Presentation Bias: This happens when visuals distort the truth. A common example is using truncated axes to exaggerate differences [1][3].
3. Interpretation Bias: This arises when data is analyzed to fit pre-existing beliefs rather than providing an objective view [1][2].
The effects of biased visuals are far-reaching. Because visuals are more memorable and shareable than text, biased infographics can spread false information, lead to bad decisions, reinforce stereotypes, and harm credibility.
Here's how the same dataset can be presented in contrasting ways:
Aspect | Biased Presentation | Unbiased Presentation |
---|---|---|
Data Source | Single group | Diverse groups |
Time Frame | Selective periods | Full timeline |
Context | Missing background info | Full context provided |
Visual Elements | Manipulated scales/axes | Accurate, standard visuals |
Recognizing these biases is the first step. Up next, we'll discuss practical strategies to reduce bias in your infographics [1][2][3].
Common Types of Bias in Infographics
Spotting the types of bias that often creep into infographics helps creators tackle potential issues before they distort the message. Let’s break down some of the most frequent examples beyond the basics.
Cherry-Picking Bias: This happens when only selective data is shown to create a favorable narrative. For instance, a 2023 sustainability infographic highlighted reduced emissions during pandemic lockdowns but failed to include the rebound to higher levels afterward [1][2].
Scale Manipulation: A classic trick where scales are adjusted to exaggerate or downplay trends. One example is a 2023 financial report that made a modest 2% market gain look comparable to a 15% increase by playing with scale proportions [2][3].
Here’s how bias often shows up in infographic design:
Bias Type | How It Appears | Impact on Perception |
---|---|---|
Visual Hierarchy Bias | Highlighting data with size or bold colors | Directs attention to specific points, ignoring others |
Temporal Bias | Using inconsistent time frames | Misleads by creating false trends |
Cultural Bias | Relying on culturally specific visuals | Excludes global audiences, limiting reach |
Statistical Bias | Misusing statistical methods | Misrepresents relationships between data |
Algorithmic Bias: As AI tools become more common in infographic creation, biases in algorithms are a growing concern. For example, facial recognition algorithms used in demographic infographics have shown higher error rates for certain ethnic groups, leading to distorted representations [2].
"Bias is a pervasive problem in AI systems, and it's not just about the data – it's about the people who design these systems." - Dr. Timnit Gebru, AI Researcher [3]
Cognitive Shortcuts Bias: This bias simplifies complex issues by relying on stereotypes. A common example is using gendered icons in workplace statistics, which reinforces outdated assumptions [1][3].
Context Omission Bias: This goes beyond leaving out data - it’s about how the missing context affects interpretation. For instance, ignoring seasonal trends or economic factors can skew the understanding of a dataset [1][2].
Understanding these biases is just the first step. The real challenge lies in applying strategies to reduce their influence on your visual content.
5 Ways to Minimize Data Bias in Infographics
Creating infographics that are fair and accurate requires careful attention to how data is collected, verified, and presented. Here are five strategies to help reduce bias while keeping your visuals clear and engaging.
1. Use Data from Multiple Sources
Pull data from a variety of sources to get a well-rounded view. Here's how you can verify data based on its origin:
Source Type | How to Verify |
---|---|
Government Data | Compare with historical records |
Academic Research | Check the credentials of the publication |
Industry Reports | Look for consistency across multiple reports |
2. Set Up Data Verification Processes
Double-check your data by cross-referencing sources and documenting how it was collected. Make sure the data covers a broad spectrum and reflects the topic accurately [1][2]. Look for patterns that might hint at bias before moving onto visualization.
3. Add Important Context
Include key details like the time period, location, and sample demographics to help your audience interpret the data correctly [1][3]. This transparency makes it clear where the data applies and where it might fall short.
4. Choose the Right Visuals
Pick chart types that best represent your data without misleading your audience [1][3]:
Data Type | Best Visual Option |
---|---|
Time Series | Line graphs to show trends over time |
Categorical | Bar charts for comparing groups |
Proportional | Pie charts to display parts of a whole |
Geographic | Maps for spatial information |
5. Conduct Routine Bias Reviews
Have a diverse group of people review your infographics, run user tests, and keep track of suggested changes [1][2]. This ongoing evaluation helps catch and fix bias throughout the design process.
1. Collect Data from a Variety of Sources
Relying on just one data source can introduce biases, like selection or confirmation bias, which can distort your visual storytelling [2]. To create well-rounded infographics, pull data from a mix of sources across different categories:
Source Type | Purpose | Example Sources |
---|---|---|
Academic Research | Peer-reviewed findings | Research journals, university studies |
Government Data | Official statistics | Census data, public health records |
Industry Reports | Market insights | Professional associations, consulting firms |
International Organizations | Global perspectives | WHO, World Bank, UNICEF |
To avoid demographic imbalances, consider oversampling underrepresented groups. For example, you might collect extra data on rural healthcare to balance urban-focused responses. Make your surveys and methods more inclusive by translating them into different languages, tailoring them to cultural contexts, and ensuring they’re accessible to all [2].
Validating Your Data
Before using data in your infographic, follow these steps to ensure its reliability:
- Cross-check information across multiple sources to confirm accuracy.
- Verify consistency in how measurements were taken.
- Ensure timelines align by checking when the data was collected.
- Document any gaps or limitations in the data for transparency.
Once you've gathered and validated diverse data, you're ready to move forward with creating an accurate and impactful infographic.
2. Check Data for Accuracy and Relevance
Making sure your data is accurate and relevant is key to creating infographics that are both reliable and engaging. The quality of your data directly affects the trustworthiness and impact of your visual storytelling.
Data Verification Framework
Verification Aspect | Key Questions | Action Steps |
---|---|---|
Source Credibility | Is the source reliable? When was it published? | Check affiliations and publication dates |
Methodology | How was the data collected? Is the sample size appropriate? | Review collection methods and sample sizes |
Data Currency | Is the information up-to-date? Are there newer datasets? | Compare with recent sources and check for updates |
Contextual Fit | Does the data match your infographic's purpose? | Analyze trends and correlations for alignment |
Quality Assurance Process
To ensure your data is reliable, focus on these key areas:
- Statistical Significance: Confirm the sample size is large enough to draw valid conclusions.
- Data Completeness: Look for missing values or gaps that could skew results.
- Consistency: Cross-check data points across different time periods and sources.
- Outlier Analysis: Investigate any unusual patterns or anomalies for potential errors.
Benchmarking and Validation
Compare your data to industry standards to spot any inconsistencies. If you're using machine learning tools or automated systems, make sure to run regular tests to catch and correct any biases [1]. This step is especially important for algorithm-based analyses.
Maintenance Schedule
To keep your data reliable, follow these review practices:
- Monthly: Check data sources for updates.
- Quarterly: Reassess the relevance of your data.
- Annually: Conduct a detailed review of methodologies.
- Ongoing: Keep an eye on industry trends to ensure your data stays current.
With your data verified and up-to-date, you're ready to focus on presenting it in a way that's clear and unbiased.
sbb-itb-3623b4a
3. Add Context to Data Points
After confirming your data's accuracy, the next step is to provide enough context to ensure clear, unbiased storytelling. Even accurate data can be misunderstood if it lacks proper context.
Key Context Types
Context Type | Purpose | Example Use |
---|---|---|
Temporal Context | Explains timeframes and trends | Highlight date ranges, seasonality, or key events |
Methodological Context | Describes how data was collected | Share survey methods, sample sizes, or limitations |
Statistical Context | Offers reference points | Use baselines, industry averages, or confidence intervals |
Demographic Context | Defines the population behind the data | Specify regions, age groups, or other segments |
How to Add Context Effectively
Make sure to clearly outline:
- Specific timeframes for data collection - avoid vague terms like "recent."
- Characteristics of your sample and any limitations.
- Methods used for measurement and how metrics were calculated.
- External factors that could influence the interpretation of the data.
Visual Tips for Context
To make your context easy to understand, use visual aids like:
- Short annotations or legends.
- Tooltips for extra information without cluttering the design.
These tools help clarify your points while keeping the visuals clean and engaging.
Mistakes to Avoid
Watch out for these common errors when adding context:
- Showing data without baseline comparisons or clear timeframes.
- Using technical jargon without explanations.
- Leaving metrics undefined or measurement methods unclear.
Double-Check Your Context
Before finalizing your infographic, ensure that all contextual details help the audience understand your data accurately. Go through each data point and confirm it answers key questions like "What?", "When?", "Where?", and "How?". This thorough review helps keep your story transparent and reduces the chance of misinterpretation [1].
The goal is to strike a balance: provide enough information for clarity while keeping your design visually appealing and easy to follow [2].
4. Use the Right Charts and Visuals
Visuals can enhance storytelling, but using them incorrectly might mislead your audience or distort the information. Picking the right charts and visuals helps ensure your infographics present data without bias.
Chart Selection Guidelines
Data Type | Recommended Charts | Best Use Case |
---|---|---|
Comparisons | Bar/Column Charts | Comparing categories or groups |
Trends | Line Charts | Tracking changes over time |
Relationships | Scatter Plots | Showing correlations |
Parts of a Whole | Bar Charts (skip pie charts for similar values) | Displaying composition |
Distribution | Histograms, Box Plots | Examining data spread |
Key Design Considerations
To make your visuals clear and effective:
- Use contrasting colors for better readability.
- Stick to consistent scales and clean, legible typography.
- Leave enough white space to avoid overwhelming the viewer [1].
Common Visualization Mistakes
Be cautious of these frequent errors:
- Using 3D charts, which can make data harder to interpret.
- Applying inconsistent scales that skew the message.
- Creating overly complicated visuals that confuse rather than clarify [1][3].
Testing Your Visuals
Before publishing your infographic, take these steps:
- Confirm that your chart type accurately represents the data.
- Double-check that the design improves understanding, not hinders it.
- Look for accessibility issues that might exclude part of your audience.
The goal is to maintain both accuracy and clarity. While aesthetics matter, they should never come at the cost of clear communication. Regular testing is your safety net for catching and fixing any potential issues [1][3].
5. Review and Test for Bias Regularly
Once you've laid the groundwork with accurate data and context, it's important to keep an eye on bias over time. Regular reviews help ensure your visuals stay fair and balanced.
Create a Review Plan
Build a systematic approach to spot and address bias:
Review Area | Testing Method | Frequency |
---|---|---|
Data Sources | Validate sources and check diversity | Before each visualization |
Algorithmic Bias | Use automated tests and manual reviews | Monthly |
Visual Elements | Get feedback from diverse focus groups | Each major update |
Audience Impact | Conduct user surveys and analyze engagement | Quarterly |
Keep Evaluating Regularly
Set up a routine to review your data and tools:
- Check how data is collected and where it comes from.
- Test your visualization tools for any unintended bias.
- Measure progress in reducing bias over time.
Collect Feedback from Different Perspectives
Engage with a variety of people to get a broader view. Consider different cultural backgrounds and levels of data literacy. Keep a record of this feedback and use it to improve future designs.
Use Specialized Tools and Methods
Apply techniques like exploratory data analysis and resampling to uncover hidden biases. Compare your findings to established benchmarks to ensure your visuals remain consistent and fair.
Track Long-term Results
Monitor how your audience interacts with your visuals. Pay attention to comprehension levels, engagement, and recurring feedback trends. This helps you understand the broader impact of your work.
Tools to Help Create Better Infographics
Designing infographics that are both accurate and balanced requires the right tools. These tools should combine features like data validation, context inclusion, and bias detection to ensure your visuals are trustworthy and effective.
Features That Minimize Bias
AI-driven tools are now equipped to catch biases early, helping you create visuals that are both accurate and impartial. For example, platforms like Text to Infographic use automated data checks and contextual analysis to address potential issues. Key features include:
Feature | How It Helps Reduce Bias |
---|---|
Source Integration | Confirms data by pulling from multiple reliable sources |
Context Analysis | Adds necessary background to avoid misrepresentation |
Bias Detection | Flags possible distortions in the data |
Verification Tools | Cross-checks data points for consistency and accuracy |
AI-Powered Bias Detection
Many modern tools now include AI capabilities designed to preserve data integrity. These features can help you:
- Integrate diverse, reliable data sources
- Use algorithms designed to avoid bias
- Monitor for inconsistencies in real time
- Perform automated checks to validate your data
Choosing the Right Tools
Look for tools that make reducing bias straightforward without complicating the design process. The best platforms combine automated features with manual control, giving you flexibility while ensuring your visuals remain accurate and engaging.
How Text to Infographic Can Help
Text to Infographic offers tools designed to improve visual storytelling while keeping bias in check. By blending AI technology with intuitive design features, the platform ensures the accuracy and integrity of data throughout the infographic creation process.
Feature | How It Addresses Bias |
---|---|
Diverse Templates | Includes designs that reflect a range of demographics without promoting stereotypes |
Multi-Source Integration | Combines and verifies data from multiple trusted sources |
Background Context | Automatically adds essential context to clarify data points |
Language Support | Allows creation in over 20 languages, making it accessible worldwide |
Additionally, users can customize their designs with brand-specific colors and logos without compromising the data's accuracy. The system ensures all edits align with strict data integrity standards, so your visuals remain both accurate and professional.
Steps to Create Accurate Infographics
Creating infographics that are both reliable and unbiased takes careful planning and regular checks. By following a structured process and using the right tools, you can produce visual content that clearly and effectively communicates your message.
Here’s a helpful framework to guide you:
Stage | Key Actions | Verification Steps |
---|---|---|
Planning | Outline how data will be collected | Identify any potential bias early |
Collection | Use a variety of data sources | Ensure data is accurate and relevant |
Analysis | Provide context for the information | Check for sampling or algorithm issues |
Design | Choose suitable visual formats | Avoid any misleading representations |
Review | Conduct thorough bias checks | Make necessary adjustments |
"To prevent bias, understand how data is created and identify issues early." [1]
Using accurate data builds trust and supports better decision-making [2]. Tools like Text to Infographic can also help streamline your design process while ensuring the data remains reliable.
To keep your infographics accurate:
- Always document and verify your data sources.
- Regularly review your content for any unintentional bias.
- Educate your team on how to spot and minimize bias in their work.
FAQs
Here are answers to some common questions about data bias in infographics.
What is an example of data bias?
Data bias happens when patterns in the data unfairly favor certain groups. A well-known example is Amazon's AI hiring tool, which was discontinued in 2018 because it relied on biased training data, leading to discriminatory outcomes. Similar biases can influence the accuracy of data-driven infographics.
How does data bias affect infographic creation?
Bias can shape how information is presented in infographics, often distorting the message. Here are two common types:
Bias Type | Example of Impact on Infographics |
---|---|
Sampling Bias | Relying only on urban data to represent an entire population. |
Selection Bias | Highlighting specific data points to misrepresent overall trends. |
Recognizing these issues is key to producing fair and accurate visuals.
How can I ensure my data sources are unbiased?
To reduce bias, check how the data was collected, compare information from multiple sources, and confirm that sample sizes reflect the full population.
What tools can help reduce data bias?
Several tools can help identify and address data bias in infographics. For instance, Text to Infographic offers features like integrating diverse data sources, analyzing context, and detecting bias in real time. However, these tools work best when paired with manual reviews to ensure the data is both accurate and fair.